The three challenges keeping cars from being fully autonomous
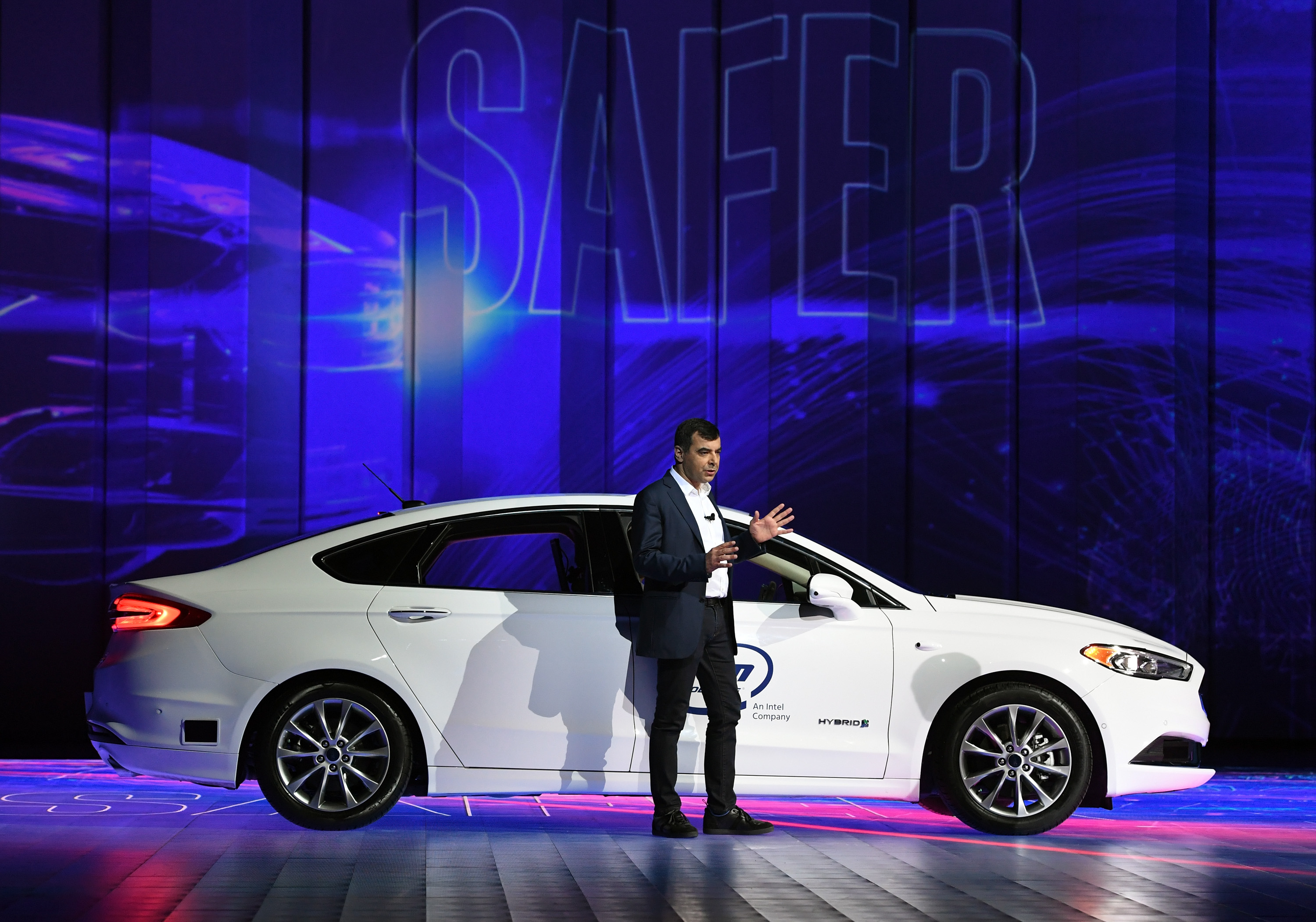
Understanding where we are in the pursuit of self-driving cars can be as confusing as understanding where we are in the pursuit of AI. Over the past few years, the flood of companies entering the space and the constant news updates have made it seem as if fully autonomous vehicles are just barely out of reach. The past couple weeks have been no different: Uber announced a new CEO and $1 billion investment for its self-driving unit, Waymo launched a ride-hailing app to open up its service to more riders in Phoenix, and Tesla unveiled a new custom AI chip that promises to unlock full autonomy.
But driverless vehicles have stayed in beta, and carmakers have wildly differing estimates of how many years we still have to go. In early April, Ford CEO Jim Hackett expressed a conservative stance, admitting that the company had initially “overestimated the arrival of autonomous vehicles.” It still plans to launch its first self-driving fleet in 2021, but with significantly dialed-back capabilities. In contrast, Tesla’s chief, Elon Musk, bullishly claimed that self-driving technology will likely be safer than human intervention in cars by 2020. “I’d be shocked if it’s not next year at the latest,” he said.
I’m not in the business of prediction. But I recently sat down with Amnon Shashua, the CEO of Mobileye, to understand the challenges of reaching full autonomy. Acquired by Intel in 2017, the Israeli-based maker of self-driving tech has partnerships with more than two dozen carmakers and become one of the leading players in the space.
Shashua presented challenges in technology, regulation, and business.
Building a safe car. From a technical perspective, Shashua splits driverless technology into two parts: its perception and its decision-making capabilities. The first challenge, he says, is to build a self-driving system that can perceive the road better than the best human driver. In the US, the current car fatality rate is about one death per 1 million hours of driving. Without drunk driving or texting, the rate probably decreases by a factor of 10. Effectively that means a self-driving car’s perception system should fail, at an absolute maximum, once in every 10 million hours of driving.
But currently the best driving assistance systems incorrectly perceive something in their environment once every tens of thousands of hours, Shashua says. “We’re talking about a three-orders-of-magnitude gap.” In addition to improving computer vision, he sees two other necessary components to closing that gap. The first is to create redundancies in the perception system using cameras, radar, and lidar. The second is to build highly detailed maps of the environment to make it even easier for a car to process its surroundings.
Building a useful car. The second challenge is to build a system that can make reasonable decisions, such as how fast to drive and when to change lanes. But defining what constitutes “reasonable” is less a technical challenge than a regulatory one, says Shashua. Anytime a driverless car makes a decision, it has to make a trade-off between safety and usefulness. “I can be completely safe if I don’t drive or if I drive very slowly,” he says, “but then I’m not useful, and society will not want those vehicles on the road.” Regulators must therefore formalize the bounds of reasonable decision-making so that automakers can program their cars to act only within those bounds. This also creates a legal framework for evaluating blame when a driverless car gets into an accident: if the decision-making system did in fact fail to stay within those bounds, then it would be liable.
Building an affordable car. The last challenge is to create a cost-effective car, so consumers are willing to switch to driverless. In the near term, with the technology still at tens of thousands of dollars, only a ride-hailing business will be financially sustainable. In that context, “you are removing the driver from the equation, and the driver costs more than tens of thousands of dollars,” Shashua explains. But individual consumers would probably not pay a premium over a few thousand dollars for the technology. In the long term, that means if automakers intend to sell driverless passenger cars, they need to figure out how to create much more precise systems than exist today at a fraction of the cost. “So the robo-taxi—we’re talking about the 2021, 2022 time frame,” he says. “Passenger cars will come a few years later.”
Mobileye is now working to overcome these challenges on all fronts. It has been refining its perception system, creating detailed road maps, and working with regulators in China, the US, Europe, and Israel to standardize the rules of autonomous driving behavior. (And it’s certainly not alone: Tesla, Uber, and Waymo are all engaging in similar strategies.) The company plans to launch a driverless robo-taxi service with Volkswagen in Tel Aviv by 2022.
This story originally appeared in our Webby-nominated AI newsletter The Algorithm. To have it directly delivered to your inbox, sign up here for free.
Deep Dive
Artificial intelligence
Large language models can do jaw-dropping things. But nobody knows exactly why.
And that's a problem. Figuring it out is one of the biggest scientific puzzles of our time and a crucial step towards controlling more powerful future models.
Google DeepMind’s new generative model makes Super Mario–like games from scratch
Genie learns how to control games by watching hours and hours of video. It could help train next-gen robots too.
What’s next for generative video
OpenAI's Sora has raised the bar for AI moviemaking. Here are four things to bear in mind as we wrap our heads around what's coming.
Stay connected
Get the latest updates from
MIT Technology Review
Discover special offers, top stories, upcoming events, and more.