First Computer to Match Humans in Conversational Speech Recognition
One by one, the skills that separate us from machines are falling into the machines’ column. First there was chess, then Jeopardy!, then Go, then object recognition, face recognition, and video gaming in general. You could be forgiven for thinking that humans are becoming obsolete.
But try any voice recognition software and your faith in humanity will be quickly restored. Though good and getting better, these systems are by no means perfect. Are you ordering “ice cream” or saying “I scream”? Probably both, if it’s a machine you are talking to.
So it ought to be reassuring to know that ordinary conversational speech recognition is something machines still struggle at—that humans are still masters of their own language.
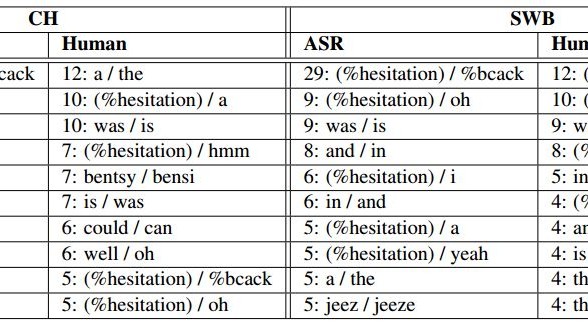
That view may have to change. Quickly. Today, Geoff Zweig and buddies at Microsoft Research in Redmond, Washington, say they’ve cracked this kind of speech recognition and that their machine-learning algorithms now outperform humans for the first time in recognizing ordinary conversational speech.
Speech recognition research has a long history. In the 1950s, early computers could recognize up to 10 words spoken clearly by a single speaker. In the 1980s, researchers built machines that could transcribe simple speech with a vocabulary of 1,000 words. In the 1990s they progressed to recordings of a person reading the Wall Street Journal, and then onto broadcast news speech.
These scenarios are all increasingly ambitious. But they are also simpler than ordinary speech because of various constraints. The vocabulary in the Wall Street Journal is limited to business and finance, and the sentences are well structured and grammatically correct, which is not necessarily true of ordinary speech. Broadcast news speech is less formal but still high structured and clearly pronounced. All of these examples have eventually been conquered by machines.
But the most difficult task—transcribing ordinary conversational speech—has steadfastly resisted the onslaught.
Ordinary speech is significantly more difficult because of the vocabulary size and also because of the noises other than words that people make when they speak. Humans use a range of noises to manage turn-taking in conversation, a type of communication that linguists call a backchannel.
For example, uh-huh is used to acknowledge the speaker and signal that he or she should keep talking. But uh is a hesitation indicating that the speaker has more to say, a warning that there is more to come. In turn management, uh plays the opposite role to uh-huh.
Humans have little difficulty parsing these sounds and understanding their role in a conversation. But machines have always struggled with them.
In 2000, the National Institute of Standards and Technology released a data set to help researchers tackle this problem. The data consisted of recordings of ordinary conversations on the telephone. Some of these were conversations between individuals on an assigned topic. The rest were conversations between friends and relatives on any topic.
Most of the data was to help train a machine-learning algorithm to recognize speech. The rest was a test that the machines had to transcribe.
The measure of performance was the number of words that the machine got wrong, and the ultimate goal was to do the task better than humans.
So how good are humans? The general consensus is that when it comes to transcription, humans have an error rate of about 4 percent. In other words, they incorrectly transcribe four words in every hundred. In the past, machines have got nowhere near this benchmark.
Now Microsoft says it has finally matched human performance, albeit with an important caveat. The Microsoft researchers began by reassessing human performance in transcription tasks. They did this by sending the telephone recordings in the NIST data set to a professional transcription service and measuring the error rate.
To their surprise, they found that this service had an error rate of 5.9 percent for the conversations between individuals on an assigned topic and 11.3 percent for the conversations between friends and family members. That’s much higher than had been thought.
Next, Zweig and co optimized their own deep-learning systems based on convolutional neural networks with varying number of layers, each of which processes a different aspect of speech. They then used the training data set to teach the machine to understand ordinary speech and let it loose on the test data set.
The results: overall, Microsoft’s speech recognition system has a similar error rate to humans, but the kinds of errors it makes are rather different.
The most common error that Microsoft machine makes is to confuse the backchannel sounds uh and uh-huh. By contrast, humans rarely make that mistake and instead tend to confuse words like a and the or uh and a.
There is no reason in principle why a machine cannot be trained to recognize backchannel sounds. Zweig and co think the difficulty the machine has with these is probably to do with the way these noises are labeled in the training data set. “The relatively poor performance of the automatic system here might simply be due to confusions in the training data annotations,” they say.
Overall, however, the machine matches the human error rate of 5.9 percent for the conversations on an assigned topic but outperforms humans in the task of transcribing friend and family conversations with an error rate of 11.1 percent. “For the first time, we report automatic recognition performance on par with human performance on this task,” say Zweig and co.
That’s interesting work. Microsoft may have moved the goalposts in recording this victory for its machines, but the writing is clearly on the wall. Machines are becoming better than humans at speech recognition. This will have significant implications for the way we interact with machines, not least when it comes to ordering ice cream.
Ref: arxiv.org/abs/1610.05256 : Achieving Human Parity in Conversational Speech Recognition
Deep Dive
Artificial intelligence
Large language models can do jaw-dropping things. But nobody knows exactly why.
And that's a problem. Figuring it out is one of the biggest scientific puzzles of our time and a crucial step towards controlling more powerful future models.
Google DeepMind’s new generative model makes Super Mario–like games from scratch
Genie learns how to control games by watching hours and hours of video. It could help train next-gen robots too.
What’s next for generative video
OpenAI's Sora has raised the bar for AI moviemaking. Here are four things to bear in mind as we wrap our heads around what's coming.
Stay connected
Get the latest updates from
MIT Technology Review
Discover special offers, top stories, upcoming events, and more.