Sponsored
Enabling enterprise growth with data intelligence
It's becoming more critical for organizations to organize data and put data infrastructure at the forefront of their data strategy, says Bharti Patel, SVP of product engineering at Hitachi Vantara.
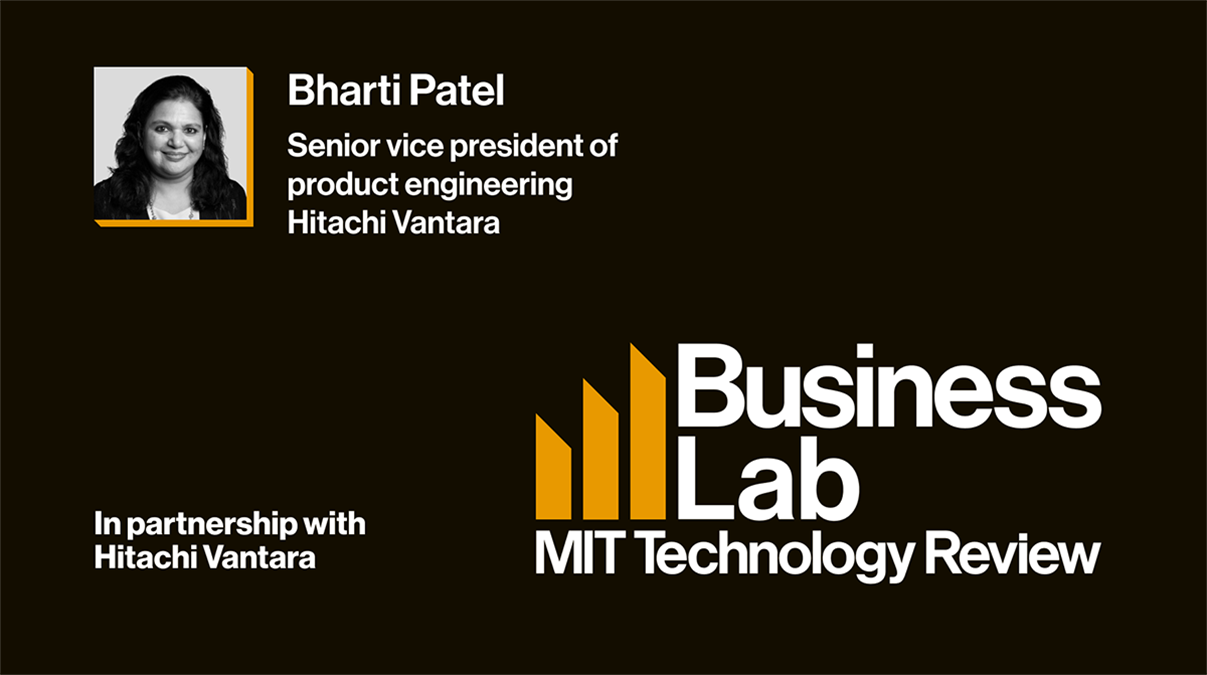
In partnership withHitachi Vantara
Data — how it’s stored and managed — has become a key competitive differentiator. As global data continues to grow exponentially, organizations face many hurdles between piling up historical data, real-time data streams from IoT sensors, and building data-driven supply chains. Senior vice president of product engineering at Hitachi Vantara, Bharti Patel sees these challenges as an opportunity to create a better data strategy.
“Before enterprises can become data-driven, they must first become data intelligent," says Patel. "That means knowing more about the data you have, whether you need to keep it or not, or where it should reside to derive the most value out of it."
Patel stresses that the data journey begins with data planning that includes all stakeholders from CIOs and CTOs to business users. Patel describes universal data intelligence as enterprises having the ability to gain better insights from data streams and meet increasing demands for transparency by offering seamless access to data and insights no matter where it resides.
Building this intelligence means building a data infrastructure that is scalable, secure, cost-effective, and socially responsible. The public cloud is often lauded as a way for enterprises to innovate with agility at scale while on premises infrastructures are viewed as less accessible and user friendly. But while data streams continue to grow, IT budgets are not and Patel notes that many organizations that use the cloud are facing cost challenges. Combating this, says Patel, means finding the best of both worlds of both on-prem and cloud environments in private data centers to keep costs low but insights flowing.
Looking ahead, Patel foresees a future of total automation. Today, data resides in many places from the minds of experts to documentation to IT support tickets, making it impossible for one person to be able to analyze all that data and glean meaningful insights.
“As we go into the future, we'll see more manual operations converted into automated operations," says Patel. "First, we'll see humans in the loop, and eventually we'll see a trend towards fully autonomous data centers."
This episode of Business Lab is produced in partnership with Hitachi Vantara.
Full transcript
Laurel Ruma: From MIT Technology Review, I'm Laurel Ruma and this is Business Lab, the show that helps business leaders make sense of new technologies coming out of the lab and into the marketplace.
Our topic today is building better data infrastructures. Doing just the basics with data can be difficult, but when it comes to scaling and adopting emerging technologies, it's crucial to organize data, tear down data silos, and focus on how data infrastructure, which is so often in the background, comes to the front of your data strategy.
Two words for you: data intelligence.
My guest is Bharti Patel. Bharti is a senior vice president of product engineering at Hitachi Vantara.
This episode of Business Lab is sponsored by Hitachi Vantara.
Welcome, Bharti.
Bharti Patel: Hey, thank you Laurel. Nice to be with you again.
Laurel: So let's start off with kind of giving some context to this discussion. As global data continues to grow exponentially, according to IDC, it's projected to double between 2022 and 2026. Enterprises face many hurdles to becoming data-driven. These hurdles include, but aren't of course limited to, piles of historical data, new real-time data streams, and supply chains becoming more data-driven. How should enterprises be evaluating their data strategies? And what are the markers of a strong data infrastructure?
Bharti: Yeah, Laurel, I can't agree more with you here. Data is growing exponentially, and as per one of the studies that we conducted recently where we talked to about 1,200 CIOs and CTOs from about 12 countries, then we have more proof for it that data is almost going to double every two to three years. And I think what's more interesting here is that data is going to grow, but their budgets are not going to grow in the same proportion. So instead of worrying about it, I want to tackle this problem differently. I want to look at how we convert this challenge into an opportunity by deriving value out of this deal. So let's talk a little more about this in the context of what's happening in the industry today.
I'm sure everyone by now has heard about generative AI and why generative AI or gen AI is a buzzword. AI has been there in the industry forever. However, what has changed recently is ChatGPT has exposed the power of AI to common people right from school going kids to grandparents by providing a very simple natural language interface. And just to talk a little bit more about ChatGPT, it is the fastest growing app in the industry. It touched 100 million users in just about two months. And what has changed because of this very fast adoption is that this has got businesses interested in it. Everyone wants to see how to unleash the power of generative AI. In fact, according to McKinsey, they're saying it's like it's going to add about $2.6 trillion to $4.4 trillion to the global economy. That means we are talking about big numbers here, but everyone's talking about ChatGPT, but what is the science behind it? The science behind it is the large language models.
And if you think of these large language models, they are AI models with billions or even trillions of parameters, and they are the science behind ChatGPT. However, to get most of these large language models or LLMs, they need to be fine-tuned because that means you're just relying on the public data. Then what you're getting, it means you're not getting first, you're not getting the information that you want, correct all the time. And of course there is a risk of people feeding bad data associated with it. So how do you make the most of it? And here actually comes your private data sets. So your proprietary data sets are very, very important here. And if you use this private data to fine-tune your models, I have no doubt in mind that it will create differentiation for you in the long run to remain competitive.
So I think even with this, we're just scratching the surface here when it comes to gen AI. And what more needs to be thought about for enterprise adoption is all the features that are needed like explainability, traceability, quality, trustworthiness, reliability. So if you again look at all these parameters, actually data is again the centerpiece of everything here. And you have to harness this private data, you have to curate it, and you have to create the data sets that will give you the maximum return on investment. Now, before enterprises can become data-driven, I think they must first become data intelligent.
And that means knowing more about the data you have, whether you need to keep it or not, or where it should reside to derive the most value out of it. And as I talk to more and more CIOs and CTOs, it is very evident that there's a lot of data out there and we need to find a way to fix the problem. Because that data may or may not be useful, but you are storing it, you are keeping it, and you are spending money on it. So that is definitely a problem that needs to be solved. Then back to your question of, what is the right infrastructure, what are some of the parameters of it? So in my mind, it needs to be nimble, it needs to be scalable, trusted, secured, cost-effective, and finally socially responsible.
Laurel: That certainly gives us a lot of perspective, Bharti. So customers are demanding more access to data and enterprises also need to get better insights from the streams of data that they're accumulating. So could you describe what universal data intelligence is, and then how it relates to data infrastructure?
Bharti: Universal data intelligence is the ability for businesses to offer seamless access to data and insights irrespective of where it resides. So basically we are talking about getting full insights into your data in a hybrid environment. Also, on the same lines, we also talk about our approach to infrastructure, which is a distributed approach. And what I mean by distributed is that you do as little data movement as possible because moving data from one place to another place is expensive. So what we are doing here at Hitachi Vantara, we are designing systems. Think of it as there is an elastic fabric that ties it all together and we are able to get insights from the data no matter where it resides in a very, very timely manner. And even this data could be in any format, from structured, unstructured, and it could be blocked to file to objects.
And just to kind of give you an example of the same, recently we worked with the Arizona Department of Water Resources to simplify their data management strategy. They have data coming from more than 300,000 water resources like means we are talking about huge data sets here. And what we did there for them was we designed an intelligent data discovery and automation tool. And in fact, we completed this data discovery and the metadata cataloging and platform migration in just two weeks with minimal downtime. And we are hearing all the time from them that they are really happy with it and they're now able to understand, integrate, and analyze the data sets to meet the needs of their water users, their planners, and their decision makers.
Laurel: So that's a great example. So data and how it's stored and managed is clearly a competitive differentiator as well. But although the amount of data is increasing, many budgets, as you mentioned, particularly IT budgets are not. So how can organizations navigate building a data infrastructure that's effective and cost-efficient? And then do you have another example of how to do more with less?
Bharti: Yeah, I think that's a great question. And this goes back to having data intelligence as the first step to becoming data-driven and reaping the full benefits of the data. So I think it goes back to you needing to know what exists and why it exists. And all of it should be available to the decision makers and the people who are working on the data at their fingertips. Just to give an example here, suppose you have data that you're just retaining because you need to just retain it for legal purposes, and the likelihood of it being used is extremely, extremely low. So there's no point in storing that data on an expensive storage device. It makes sense to transfer that data to a low cost object storage.
And at the same time, you might have the data that you need to access all the time. And speed is important. Low latency is important, and that kind of data needs to reside on fast NVMEs. And in fact, many of our customers do it all the time, and in fact in all the sectors. So what they do is they have their data, which through the policies, they constantly transfer from our highly, highly efficient file systems to object storage based on the policies. And it's like they still retain the pointers there in the file system and they're able to access it back in case they need it.
Laurel: So the public cloud is often cited as a way for enterprises to scale, be more agile, and innovate while by contrast, legacy on-premises infrastructures are seen as less user-friendly and accessible. How accurate is this conception and how should enterprises approach data modernization and management of that data?
Bharti: Yeah, I've got to admit here that the public cloud and the hyperscalers have raised the bar in terms of what is possible when it comes to innovation. However, we are also seeing and hearing from our customers that the cost is a concern there. And in fact, many of our customers, they move to cloud very fast and now they're facing the cost challenge. When their CIOs see the bills going exponentially up, they're asking like, "Hey, well how could we keep it flat?" That's where I think we see a big opportunity, how to provide the same experience that cloud provides in a private data center so that when customers are talking about partition of the data, we have something equivalent to offer.
And here again, I have got to say that we want to address in a slightly different manner. I think we want to address it so that customers are able to take full advantage of the elasticity of the cloud, and also they're able to take full advantage of on-prem environments. And how we want to do it, we want to do it in such a way that it's almost in a seamless way, in a seamless manner. They can manage the data from their private data centers, doing the cloud and get the best from both worlds.
Laurel: An interesting perspective there, but this also kind of requires different elements of the business to come in. So from a leadership perspective, what are some best practices that you've instituted or recommended to make that transition to better data management?
Bharti: Yeah, I would say I think the data journey starts with data planning, and which should not be done in a siloed manner. And getting it right from the onset is extremely, extremely important. And what you need to do here is at the beginning of your data planning, you've got to get all the stakeholders together, whether it's your CIO, your business users, your CTOs. So this strategy should never be done in a siloed manner. And in fact, I do want to think about, highlight another aspect, which probably people don't do very much is how do you even bring your partners into the mix? In fact, I do have an example here. Prior to joining Hitachi Vantara, I was a CTO, an air purifier company. And as we were defining our data strategy, we were looking at our Salesforce data, we were looking at data in our NetSuite, we were looking at the customer tickets, and we were doing all this to see how we can drive marketing campaigns.
And as I was looking at this data, I felt that something was totally missing. And in fact, what was missing was the weather data, which is not our data, which was third-party data. For us to design effective marketing campaigns, it was very important for us to have insights into this weather data. For example, if there are allergies in a particular region or if there are wildfires in a particular region. And that data was so important. So having a strategy where you are able to bring all stakeholders, all parts of data together and think from the beginning is the right thing to get started.
Laurel: And with big hairy problems and goals, there's also this consideration that data centers contribute to an enterprise's carbon emissions. Thinking about partnerships and modernizing data management and everything we've talked about so far, how can enterprises meet sustainability goals while also modernizing their data infrastructure to accommodate all of their historical and real-time data, especially when it comes from, as you mentioned, so many different sources?
Bharti: Yeah, I'm glad that you are bringing up this point because it's very important not to ignore this. And in fact, with all the gen AI and all the things that we are talking about, like one fine-tuning of one model can actually generate up to five times the carbon emissions that are possible from a passenger car in a lifetime. So we're talking about a huge, huge environmental effect here. And this particular topic is extremely important to Hitachi. And in fact, our goal is to go carbon-neutral with our operations by 2030 and across our value chain by 2050. And how we are addressing this problem here is kind of both on the hardware side and also on the software side. Right from the onset, we are designing our hardware, we are looking at end-to-end components to see what kind of carbon footprint it creates and how we could really minimize it. And in fact, once our hardware is ready, actually, it needs to pass through a very stringent set of energy certifications. And so that's on the hardware side.
Now, on the software side, actually, I have just started this initiative where we are looking at how we can move to modern languages that are more likely to create less carbon footprint. And this is where we are looking at how we can replace our existing Java [code base] with Rust, wherever it makes sense. And again, this is a big problem we all need to think about and it cannot be solved overnight, but we have to constantly think about interface manner.
Laurel: Well, certainly are impressive goals. How can emerging technologies like generative AI, as you were saying before, help push an organization into a next generation of data infrastructure systems, but then also help differentiate it from competitors?
Bharti: Yeah, I want to take a kind of a two-pronged approach here. First, what I call is table stakes. So if you don't do it, you'll be completely wiped out. And these are simple things about how you automate certain things, how you create better customer experience. But in my mind, that's not enough. You got to think about what kind of disruptions you will create for yourself and for your customers. So a couple of ideas that we are working on here are the companions or copilots. And these are, think of them as AI agents in the data centers. And these agents actually help the data center environment from becoming more reactive to proactive.
So basically these agents are running in your data center all the time and they're watching if there is a new patch available and if you should update to the new patch, or maybe there's a new white paper that has better insights to manage some of your resources. So this is like these agents are constantly acting in your data center. They are aware of what's going on on the internet based on how you have designed, and they're able to provide you with creative solutions. And I think that's going to be the disruption here, and that's something we are working on.
Laurel: So looking to the future, what tools, technologies, or trends do you see emerging as more and more enterprises look to modernize their data infrastructure and really benefit from data intelligence?
Bharti: Again, I'll go back to what I'm talking about, generative AI here, and I'll give an example. For one of our customers, we are managing their data center, and I'm also part of that channel where we see constant back and forth between the support and the engineering. The support is asking, "Hey, this is what is happening, what should we be doing?" So just think of it like a different scenario that you have all this and you were able to collect this data and feed it into the LLMs. When you're talking about this data, this data resides at several places. It resides in the heads of our experts. It is there in the documentation, it's there in the support tickets, it's there in logs, like life logs. It is there in the traces. So it's almost impossible for a human being to analyze this data and get meaningful insights.
However, if we combine LLMs with the power of, say, knowledge graphs, vector databases, and other tools, it will be possible to analyze this data at the speed of light, and present the recommendation in front of the user through a very simple user interface. And in most cases, just via a very simple natural language interface. So I think that's a kind of a complete paradigm shift where you have so many sources that you need to constantly analyze versus having the full automation. And that's why I feel that these copilots will become an essential part of the data centers. In the beginning they'll help with the automation to deal with the problems prevalent in any data center like resource management and optimization, proactive problem determination, and resolution of the same. As we go into the future, we'll see more manual operations converted into automated operations. First, we'll see humans in the loop, and eventually we'll see a trend towards fully autonomous data centers.
Laurel: Well, that is quite a future. Thank you very much for joining us today on the Business Lab.
Bharti: Thank you, Laurel. Bye-bye.
Laurel: That was Bharti Patel, who is the senior vice president of Product Marketing at Hitachi Vantara who I spoke with from Cambridge, Massachusetts, the home of MIT and MIT Technology Review.
That's it for this episode of Business Lab. I'm your host, Laurel Ruma. I'm the director of Insights, the custom publishing division of MIT Technology Review. We were founded in 1899 at the Massachusetts Institute of Technology, and you can find us in print, on the web, and at events each year around the world. For more information about us and the show, please check out our website at technologyreview.com.
This show is available wherever you get your podcasts. If you enjoyed this episode, we hope you'll take a moment to rate and review us. Business Lab is a production of MIT Technology Review. This episode was produced by Giro Studios. Thanks for listening.
This content was produced by Insights, the custom content arm of MIT Technology Review. It was not written by MIT Technology Review’s editorial staff.
Deep Dive
Humans and technology
Building a more reliable supply chain
Rapidly advancing technologies are building the modern supply chain, making transparent, collaborative, and data-driven systems a reality.
Building a data-driven health-care ecosystem
Harnessing data to improve the equity, affordability, and quality of the health care system.
Let’s not make the same mistakes with AI that we made with social media
Social media’s unregulated evolution over the past decade holds a lot of lessons that apply directly to AI companies and technologies.
Stay connected
Get the latest updates from
MIT Technology Review
Discover special offers, top stories, upcoming events, and more.