Machine vision has learned to use radio waves to see through walls and in darkness
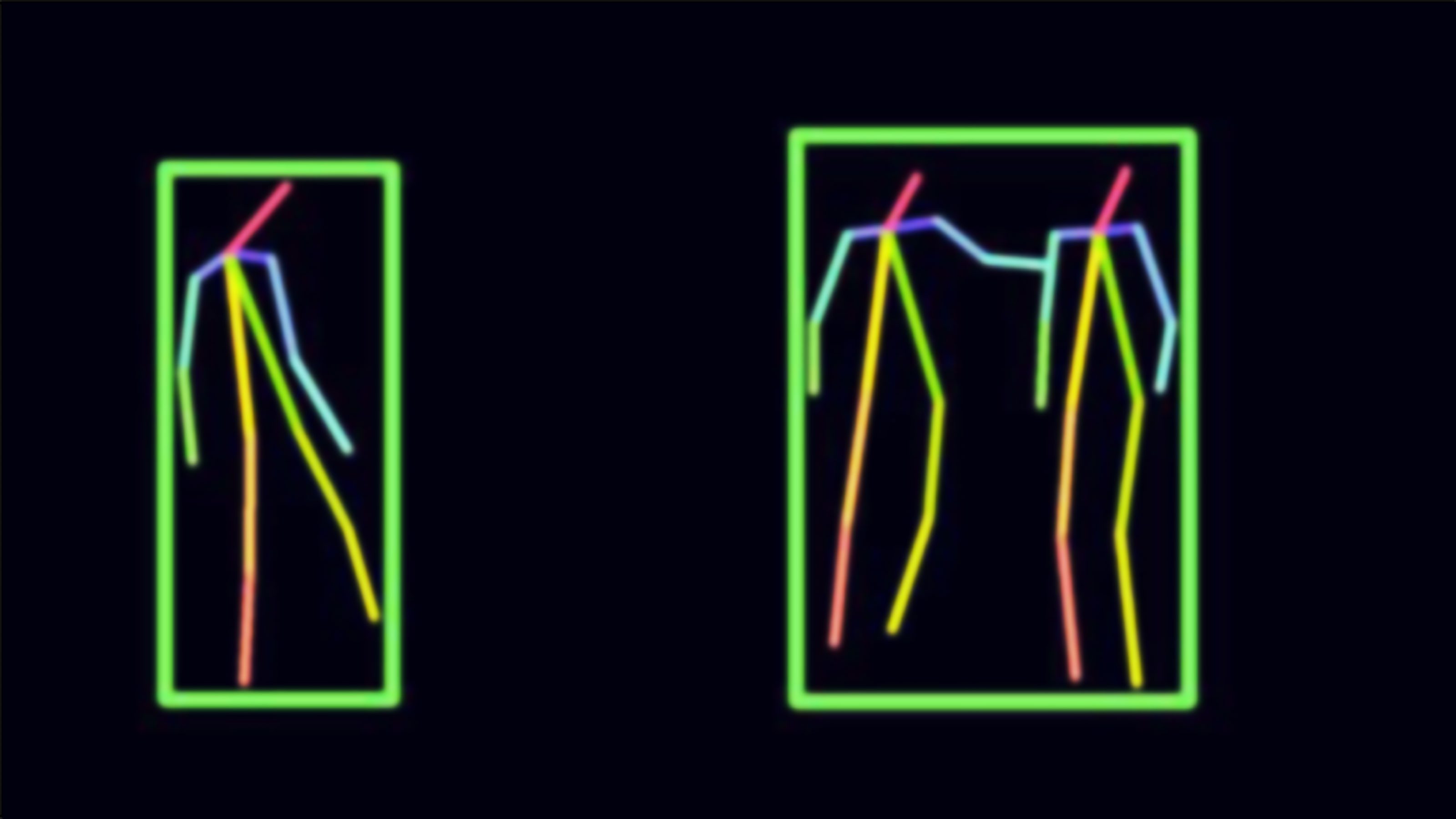
Machine vision has an impressive record. It has the superhuman ability to recognize people, faces and objects. It can even recognize many different kinds of actions, albeit not quite as well as humans just yet.
But there are limits to its performance. Machines have a particularly difficult time when people, faces, or objects are partially occluded. And when light levels drop too far, they are effectively blinded, just like humans.
But there is another part of the electromagnetic spectrum that is not limited in the same way. Radio waves fill our world, whether it is night or day. They pass easily through walls and are both transmitted and reflected by human bodies. Indeed, researchers have developed various ways to use Wi-Fi radio signals to see behind closed doors.
But these radio vision systems have some shortcomings. Their resolution is low; the images are noisy and filled with distracting reflections, which make it hard to make sense of what’s going on.
In this sense, radio images and visible-light images have complementary advantages and disadvantages. And that raises the possibility of using the strengths of one to overcome the shortcomings of the other.
Enter Tianhong Li and colleagues at MIT, who have found a way to teach a radio vision system to recognize people’s actions by training it with visible-light images. The new radio vision system can see what individuals are up to in a wide range of situations where visible-light imaging fails. “We introduce a neural network model that can detect human actions through walls and occlusions, and in poor lighting conditions,” say Li and co.
The team’s method uses a neat trick. The basic idea is to record video images of the same scene using visible light and radio waves. Machine-vision systems are already able to recognize human actions from visible-light images. So the next step is to correlate those images with the radio images of the same scene.
But the difficulty is in ensuring that the learning process focuses on human movement rather than other features, such as the background. So Li and co introduce an intermediate step in which the machine generates 3D stick-figure models that reproduce the actions of the people in the scene.
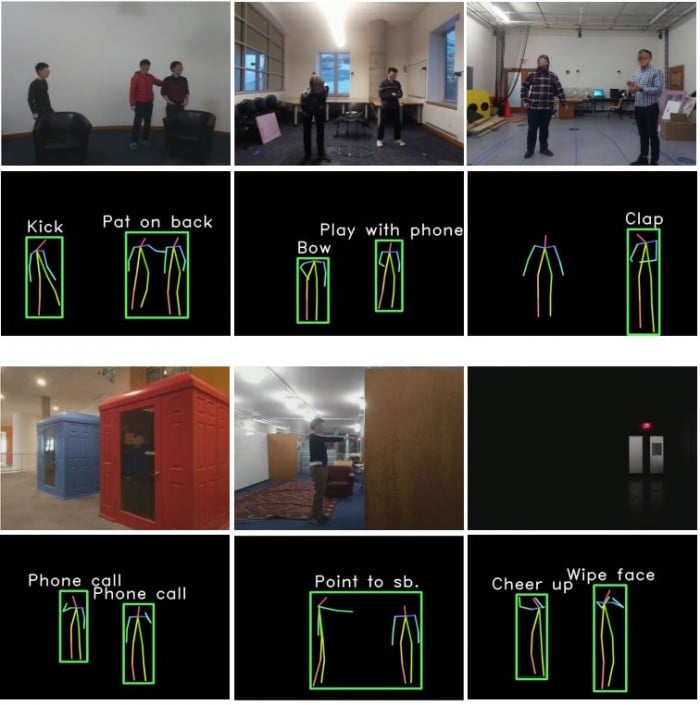
”By translating the input to an intermediate skeleton-based representation, our model can learn from both vision-based and radio frequency-based datasets, and allow the two tasks to help each other.” say Li and co.
In this way the system learns to recognize actions in visible light and then to recognize the same actions taking place in the dark or behind walls, using radio waves. “We show that our model achieves comparable accuracy to vision-based action recognition systems in visible scenarios, yet continues to work accurately when people are not visible,” say the researchers.
That’s interesting work that has significant potential. The obvious applications are in scenarios where visible-light images fail—in low light conditions and behind closed doors.
But there are other applications too. One problem with visible-light images is that people are recognizable, which raises privacy issues.
But a radio system does not have the resolution for facial recognition. Identifying actions without recognizing faces does not raise the same privacy fears. “It can bring action recognition to people’s homes and allow for its integration in smart home systems,” say Li and co. That could be used to monitor an elderly person’s house and alert the appropriate services about a fall, for example. And it would do so without much risk to privacy.
That’s beyond the capability of today’s vision-based systems.
Ref: arxiv.org/abs/1909.09300 : Making the Invisible Visible: Action Recognition Through Walls and Occlusions
Deep Dive
Humans and technology
Building a more reliable supply chain
Rapidly advancing technologies are building the modern supply chain, making transparent, collaborative, and data-driven systems a reality.
Building a data-driven health-care ecosystem
Harnessing data to improve the equity, affordability, and quality of the health care system.
Let’s not make the same mistakes with AI that we made with social media
Social media’s unregulated evolution over the past decade holds a lot of lessons that apply directly to AI companies and technologies.
Stay connected
Get the latest updates from
MIT Technology Review
Discover special offers, top stories, upcoming events, and more.