AI Programs Are Learning to Exclude Some African-American Voices
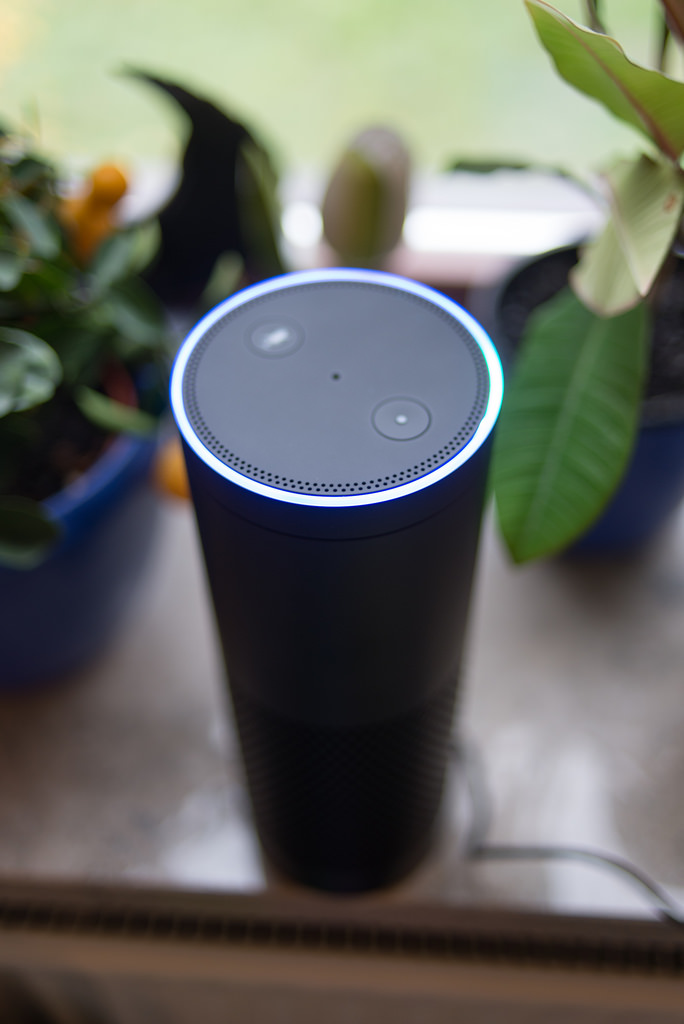
All too often people make snap judgments based on how you speak. Some AI systems are also learning to be prejudiced against some dialects. And as language-based AI systems become ever more common, some minorities may automatically be discriminated against by machines, warn researchers studying the issue.
Anyone with a strong or unusual accent may know what it’s like to have trouble being understood by Siri or Alexa. This is because voice-recognition systems use natural-language technology to parse the contents of speech, and it often relies on algorithms that have been trained with example data. If there aren’t enough examples of a particular accent or vernacular, then these systems may simply fail to understand you (see “AI’s Language Problem”).
The problem may be more widespread and pernicious than most people realize. Natural-language technology now powers automated interactions with customers, through automated phone systems or chatbots. It’s used to mine public opinion on the Web and social networks, and to comb through written documents for useful information. This means that services and products built on top of language systems may already be unfairly discriminating against certain groups.
Brendan O’Connor, an assistant professor at the University of Massachusetts, Amherst, and one of his graduate students, Su Lin Blodgett, looked at the use of language on Twitter. Using demographic filtering, the researchers collected 59.2 million tweets with a high probability of containing African-American slang or vernacular. They then tested several natural-language processing tools on this data set to see how they would treat these statements. They found that one popular tool classified these posts as Danish with a high level of confidence.
“If you analyze Twitter for people’s opinions on a politician and you’re not even considering what African-Americans are saying or young adults are saying, that seems problematic,” O’Connor says.
The pair also tested several popular machine-learning-based APIs that analyze text for meaning and sentiment, and they found that these systems struggled, too. “If you purchase a sentiment analyzer from some company, you don’t even know what biases it has in it,” O’Connor says. “We don’t have a lot of auditing or knowledge about these things.”
He says the problem extends to any system that uses language, including search engines.
The issue of unfairness emerging from the use of AI algorithms is gaining attention in some quarters as these algorithms are used more widely. One controversial example of possible bias is a proprietary algorithm called Compass, which is used to decide whether prison inmates should be granted parole. The workings of the algorithm are unknown, but research suggests it is biased against black inmates.
Some experts, however, say that the problem may be more serious than many people know, affecting a growing number of decisions in finance, health care, and education (see “Biased Algorithms are Everywhere and No One Seems to Care”).
The UMass researchers presented their work at a workshop dedicated to exploring the issue of bias in AI. The event, Fairness and Transparency in Machine Learning, was part of a larger data-science conference this year, but it will become a stand-alone conference itself in 2018. Solon Barocas, an assistant professor at Cornell and a cofounder of the event, says the field is growing, with more and more researchers exploring the issue of bias in AI systems.
Shared Goel, an assistant professor at Stanford University who studies algorithmic fairness and public policy, says the issue is not always straightforward. He notes that it can be overly simplistic to call algorithms biased, in that they may work entirely as intended, making predictions that are accurate, and simply reflect broader social biases. “It’s better to describe what an algorithm is doing, the reason it’s doing it, and then to decide if that’s what we want it to do,” Goel says.
Keep Reading
Most Popular
Large language models can do jaw-dropping things. But nobody knows exactly why.
And that's a problem. Figuring it out is one of the biggest scientific puzzles of our time and a crucial step towards controlling more powerful future models.
How scientists traced a mysterious covid case back to six toilets
When wastewater surveillance turns into a hunt for a single infected individual, the ethics get tricky.
The problem with plug-in hybrids? Their drivers.
Plug-in hybrids are often sold as a transition to EVs, but new data from Europe shows we’re still underestimating the emissions they produce.
Stay connected
Get the latest updates from
MIT Technology Review
Discover special offers, top stories, upcoming events, and more.