Back in 2013, a handful of researchers at Google set loose a neural network on a corpus of three million words taken from Google News texts. The neural net’s goal was to look for patterns in the way words appear next to each other.
What it found was complex but the Google team discovered it could represent these patterns using vectors in a vector space with some 300 dimensions.
It turned out that words with similar meanings occupied similar parts of this vector space. And the relationships between words could be captured by simple vector algebra. For example, “man is to king as woman is to queen” or, using the common notation, “man : king :: woman : queen.” Other relationships quickly emerged too such as “sister : woman :: brother : man,” and so on. These relationships are known as word embeddings.
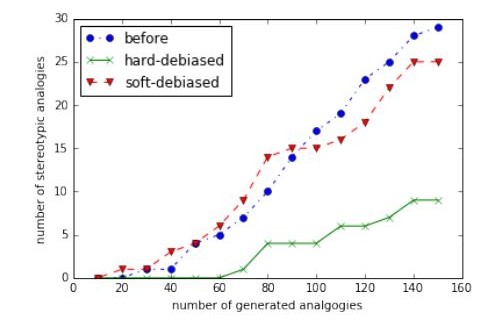
This data set is called Word2vec and is hugely powerful. Numerous researchers have begun to use it to better understand everything from machine translation to intelligent Web searching.
But today Tolga Bolukbasi at Boston University and a few pals from Microsoft Research say there is a problem with this database: it is blatantly sexist.
And they offer plenty of evidence to back up the claim. This comes from querying the vector space to find word embeddings. For example, it is possible to pose the question: “Paris : France :: Tokyo : x” and it will give you the answer x = Japan.
But ask the database “father : doctor :: mother : x” and it will say x = nurse. And the query “man : computer programmer :: woman : x” gives x = homemaker.
In other words, the word embeddings can be dreadfully sexist. This happens because any bias in the articles that make up the Word2vec corpus is inevitably captured in the geometry of the vector space. Bolukbasi and co despair at this. “One might have hoped that the Google News embedding would exhibit little gender bias because many of its authors are professional journalists,” they say.
So what to do? The Boston team has a solution. Since a vector space is a mathematical object, it can be manipulated with standard mathematical tools.
The solution is obvious. Sexism can be thought of as a kind of warping of this vector space. Indeed, the gender bias itself is a property that the team can search for in the vector space. So fixing it is just a question of applying the opposite warp in a way that preserves the overall structure of the space.
That’s the theory. In practice, the tricky part is measuring the nature of this warping. The team does this by searching the vector space for word pairs that produce a similar vector to “she: he.” This reveals a huge list of gender analogies. For example, she;he::midwife:doctor; sewing:carpentry; registered_nurse:physician; whore:coward; hairdresser:barber; nude:shirtless; boobs:ass; giggling:grinning; nanny:chauffeur, and so on.
The question they want to answer is whether these analogies are appropriate or inappropriate. So they use Amazon’s Mechanical Turk to ask. They showed each analogy to 10 turkers and asked them whether the analogy was biased or not. They consider the analogy biased if more than half of the turkers thought it was biased.
The results make for interesting reading. This method clearly reveals a gender bias in pairings such as midwife:doctor; sewing:carpentry, and registered_nurse:physician, but that there is little bias in pairings such as feminine:manly; convent:monastery; handbag:briefcase, and so on.
Having compiled a comprehensive list of gender biased pairs, the team used this data to work out how it is reflected in the shape of the vector space and how the space can be transformed to remove this warping. They call this process “hard de-biasing.”
Finally, they use the transformed vector space to produce a new list of gender analogies and then ask turkers to rate them again. This produces pairings such as: she:he::hen:cock; maid:housekeeper; gals:dudes; daughter:son, and so on.
This process, they say, dramatically reduces the bias that Turkers report. “Through empirical evaluations, we show that our hard-debiasing algorithm significantly reduces both direct and indirect gender bias while preserving the utility of the embedding,” say Bolukbasi and co.
The end result is a vector space in which the gender bias is significantly reduced.
That has important applications. Any bias contained in word embeddings like those from Word2vec is automatically passed on in any application that exploits it. One example is the work using embeddings to improve Web search results. If the phrase “computer programmer” is more closely associated with men than women, then a search for the term “computer programmer CVs” might rank men more highly than women. “Word embeddings not only reflect stereotypes but can also amplify them,” say Bolukbasi and co.
Clearly, language is filled with many examples of gender bias that are hard to justify. An interesting question is the extent to which this kind of vector space mathematics should be used to correct it.
“One perspective on bias in word embeddings is that it merely reflects bias in society, and therefore one should attempt to debias society rather than word embeddings,” say Bolukbasi and co. “However, by reducing the bias in today’s computer systems (or at least not amplifying the bias), which is increasingly reliant on word embeddings, in a small way debiased word embeddings can hopefully contribute to reducing gender bias in society.”
That seems a worthy goal. As the Boston team concludes: “At the very least, machine learning should not be used to inadvertently amplify these biases.”
Ref: arxiv.org/abs/1607.06520: Man Is to Computer Programmer as Woman is to Homemaker? Debiasing Word Embeddings.
Deep Dive
Space
How to safely watch and photograph the total solar eclipse
The solar eclipse this Monday, April 8, will be visible to millions. Here’s how to make the most of your experience.
The great commercial takeover of low Earth orbit
Axiom Space and other companies are betting they can build private structures to replace the International Space Station.
How scientists are using quantum squeezing to push the limits of their sensors
Fuzziness may rule the quantum realm, but it can be manipulated to our advantage.
Stay connected
Get the latest updates from
MIT Technology Review
Discover special offers, top stories, upcoming events, and more.