Sponsored
Purpose-built AI builds better customer experiences
Successfully improving customer satisfaction through AI means becoming data-driven, prioritizing employee feedback and resources, and letting business goals guide technology deployment, says senior product marketing manager at NICE, Michele Carlson.
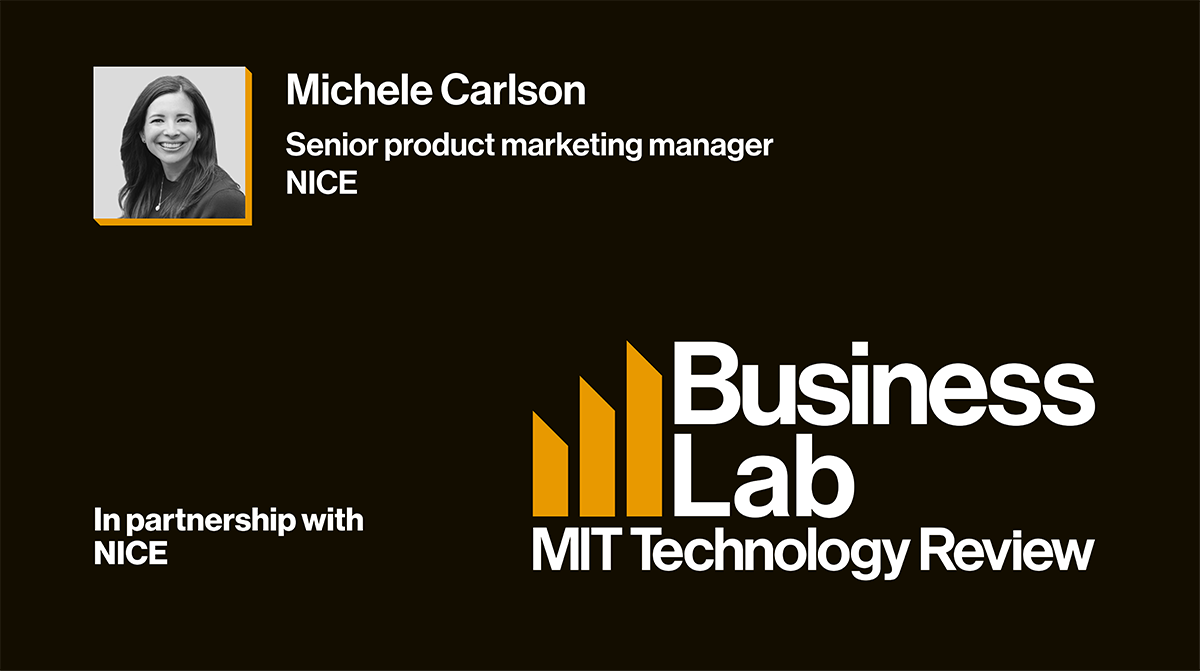
In partnership withNICE
In the bygone era of contact centers, the customer experience was tethered to a singular channel—the phone call. The journey began with a pre-recorded message prompting the customer to press a number corresponding to their query. Today's contact centers have evolved from the confines of just traditional phone calls to multiple channels from emails to social media to chatbots.
Customers have access to more business information than ever. But improving the quality of customer experiences means becoming more customer-centric and data-driven and scaling available human representatives for round-the-clock assistance.
Enabling these improvements is no small feat for enterprises, though, says senior product marketing manager at NICE, Michele Carlson. With large data streams and the demand for personalized experiences, artificial intelligence has become the key enabler in fostering these better customer experiences.
"There's such an enormous amount of data available that without artificial intelligence as this driving force for better customer experiences, it would be impossible to meet customer's expectations today."
Amid the many moving parts in a contact center from managing multiple incoming calls to taking accurate notes of each interaction to measuring success metrics, AI can help smooth friction. Sentiment analysis can help supervisors identify in real-time which calls require escalation or further support and AI tools can summarize calls and automate note-taking to free up agents to focus more closely on customer needs. These use cases not only improve customer and employee experiences but also save time and money.
While the promises of AI have many enterprises making swift investments, Carlson cautions leaders to be goal-oriented first. Rather than deploy AI because it's popular, AI-driven solutions need to be purpose-built to support and align with goals.
"There are so many available artificial intelligence solutions right now, but it's really critical to choose AI that is designed and built on data that is specific to your organization," says Carlson.
Looking ahead, Carlson sees the evolution toward AI-enabled customer centricity as a signal of a customer experience paradigm shift where AI will augment not just operational details but offer insights into high-level business strategy.
"As everyone gets introduced to this technology," says Carlson, "it's going to be those that are open to using new things and open to using AI, but also the ones that are selecting the right types of artificial intelligence to compliment their business that are going to be the most successful in using it, and gaining the efficiency and optimizing the customer experiences."
This episode of Business Lab is produced in partnership with NICE.
Full Transcript
Laurel Ruma: From MIT Technology Review, I'm Laurel Ruma, and this is Business Lab, the show that helps business leaders make sense of new technologies coming out of the lab and into the marketplace.
Our topic is deploying customer service with AI to maximize results. As artificial intelligence evolves in the call center, it can provide real-time guidance. But measuring success remains key to operational efficiency and customer satisfaction.
Two words for you: better service.
My guest is Michele Carlson, senior product marketing manager at NICE.
This podcast is produced in partnership with NICE.
Welcome, Michele.
Michele: Thank you so much, Laurel. I'm so excited to be here.
Laurel: Well, welcome. And let's begin by setting some context for our conversation. Long ago, in technology years, one would talk to a live person when calling a customer service number, and then we moved on to automated menu choices and beyond. So how have call centers evolved to better serve customers? And to bring us up to the present day, how is AI an enabler of that evolution?
Michele: The really good place to get started is, how did this all begin? So right now, contact centers are more customer-focused than they've ever been. Like you mentioned, they first started with a call, or maybe what we call an IVR, or an internal voice recording, where you would put in a phone number, or put in a number if you wanted to go to a certain queue to answer a certain type of question. And now we've advanced far beyond that.
So there still are things like IVRs in the market, but there are more channels than ever now that customers are interacting with. So it's not just the phone calls, it's email, it's social, it's the chatbots on their website. It's the more sophisticated website. So there's more places that customers can get information about a business than ever before. So that's something that's really changed for contact centers.
So the way that they're really handling that to give better customer experience, and to engage more with their customers, is focusing more on becoming customer-centric. Which are things like more personalization, being more data-driven, having greater availability for their agents. And all of these options that, for us as consumers, are really exciting because we can reach out to a business in many different ways at many different hours of the day, 24/7 access to get our questions answered.
While this is exciting for customers, it also creates a challenge for contact centers. Because, yes, it's a way that they can evolve to serve their customers in all these places, but it's a challenge for them. And you asked about artificial intelligence or AI, how is AI supporting that?
And that's a big enabler for contact centers to be able to deliver these better experiences to customers, because there are so many channels, there's so much need and expectation for personalization. There is a need to be more data-driven. And artificial intelligence allows businesses, allows contact centers, to evaluate and see what their customers are calling about, when their customers are going to call, what channels their customers are interacting with, and even the questions that customers are asking on different channels.
Using all of that data is a way that they can personalize and deliver better experiences. And artificial intelligence allows them to look at all that data. There's such an enormous amount of data available that without artificial intelligence as this driving force for better customer experiences, it would be impossible to meet customer's expectations today.
And so it's really exciting to think ... As you mentioned, it's a long time ago in technology years, which is really a very short time. We've seen this evolution really pick up pace in the last few years with the integration of things like conversational AI and generative AI into that contact center space. And we'll talk more about those in the course of our conversation, too.
Laurel: So yeah, speaking of data, it's such a central role to most technology deployments and digital transformations. So then, what is the role of data in this context? And how can organizations best manage and use the data, since it is coming from so many different places as well as where it needs to be saved, to ensure a more efficient experience with contact centers?
Michele: Yeah, so the role data plays in our world today is a substantial one. “Data is the new oil.” It's not my quote, but I'll borrow it.
And data, there is so much of it. And the idea is it's so very valuable, and it's really critical to have all this data gathered together to be able to use it and be able to understand it.
So what contact centers are doing, the ones that are really successful in this, is they're benefiting by aligning their data and building what we're calling an interaction-centric approach.
Rather than saying I'm just going to look at my customers in a web version, or I'm just going to look at my customers through voice, being able to look at data from all over and all these different places makes this interaction-centric approach really crucial to getting started and using the data in a way that makes sense for the business.
So this is allowing them to move from things like voice and digital messaging to chatbots and social media, just on one platform. So if you or me, if we were to call into a contact center, they would know where our journey has gone. If we went to the website, if we went to the chatbot, if we called, how our call went, who we spoke with, what the outcome of that interaction was.
And that lens, in having the data, is more powerful in keeping this customer-centric approach, or this customer-centric mindset. Because it brings together all of these touch points on one channel, so that you can move interactions into one platform, which allows all these organizations to then look at different types of applications and solutions to solve different problems within their contact centers and their customer experience groups.
Laurel: So could you share some of those specific examples of how AI-driven solutions can address these unique challenges in contact centers, and also provide improvements in both customer and employee experiences?
Michele: Yes, of course. And I really like how you frame that question. Because it's about both the customer experience and the employee experience. Without helping your employees and supporting your employees, it would be very difficult to provide, in turn, that great customer experience. And artificial intelligence-driven, AI-driven types of solutions, just to go back to that previous question around data, the AI solutions are only as good as the data that's available to them.
So in a contact center where customer experience is the goal, you want your artificial intelligence and the data to be driven off of interactions with your customers, and that's a very crucial foundational element across the board in choosing and using an artificially intelligent solution. One of the ways that organizations are doing this, they're thinking about, we started with that IVR [interactive voice response]. By the time I get to item nine in the menu, I've usually forgotten what the previous items are.
But rather than using an IVR, you can use artificially intelligent routing. So you can predict why a customer is calling, who and which agent they might best interact with. And you can use data kind of on both sides to understand the customer's needs, and the agents, to direct the call so it has the best outcome.
Once the interaction begins, we can use data, artificial intelligence, to measure sentiment, customer sentiment. And in the course of the interaction, an agent can get a notification from their supervisor that says, "Here's a couple different things that you can do to help improve this call." Or, "Hey, in our coaching session, we talked about being more empathetic, and that's what this means for this customer." So, giving specific prompts to make the interaction move better in real-time.
Another example supervisors are also burdened with; they usually have a large team of somewhere up to 20, sometimes 25 different agents who all have calls going at the same time.
And it's difficult for supervisors to keep a pulse on, who is on which interaction with what customer? And is this escalation important, or which is the most important place? Because we can only be one place at one time. As much as we try with modern technology to do many things, we can only do one really well at once.
So for supervisors, they can get a notification about which calls are in need of escalation, and where they can best support their agent. And they can see how their teams are performing at one time as well.
Once the call is over, artificial intelligence can do things like summarize the interaction. During a context interaction, agents take in a lot of information. And it is difficult to then decipher that, and their next call is going to be coming in very quickly. So artificial intelligence can generate a summary of that interaction, instead of the agent having to write notes.
And this is a huge improvement because it improves the experience for customers. That next time they call, they know those notes are going to go over to the agent, the agent can use them. Agents also really appreciate this, because it's difficult for them in shorthand to recreate very complicated, in healthcare for example, all of the different coding numbers for different types of procedures, or are the provider, or multiple providers, or explanations of benefits to summarize all of that concisely before they take their next call.
So an auto-summarization tool does that automatically based off of the conversation, saving the agents up to a minute of post-call notes, but also saving businesses upwards of $14 million a year for 1,000 agents. Which is great, but agents appreciate it because 85% of them don't really like all of their desktop applications. They have a lot of applications that they manage. So artificial intelligence is helping with these call summaries.
It can also help with reporting after the fact, to see how all of the calls are trending, is there high sentiment or low sentiment? And also in the quality management aspect of managing a contact center, every single call is evaluated for compliance, for greeting, for how the agent resolved the call. And one of the big challenges in quality management without artificial intelligence is that it's very subjective.
So you and I could listen to the same call, and we could have very different viewpoints of how the call went. And agents, it's difficult for them to get conflicting feedback on their performance. And so artificial intelligence can listen to the call, extract data points baseline, and consistently evaluate every single interaction that's coming into a contact center.
They get better feedback and then they grow, they learn, they have a better overall experience because of this consistency in the evaluations.
So to answer your question, there are a lot of different ways artificial intelligence can support these contact center needs. And if you're a business and customer satisfaction is your main goal, it's really critical to think about not just one point of an interaction you have with a customer, but really before, during, and after every interaction, there's all these opportunities to bring in data for greater consistency, and that's something that is gained through using artificial intelligence.
Laurel: Yeah, that's certainly quite a bit there. So when a company is thinking about integrating AI into their customer experiences, what are some common pitfalls they need to look out for, and how can those be mitigated or avoided?
Michele: Yeah, I think one of the most common pitfalls, and we're all attracted to what's new and exciting, and artificial intelligence is definitely on that list. And one of the reasons, or one of the pitfalls I've seen as organizations are getting started, they focus on too much on using AI.
Somebody said they read a cool article, "We've got to use AI for that." And yeah, you could use AI for that. But really you're choosing a type of technology, or you're choosing artificial intelligence, to solve a specific problem. So what I would encourage everyone to do is, think about what is your goal? And then choose AI-driven solutions to then support and align with your goals.
So for typical goals in the contact center, these might be around measuring customer experience like CSAT, sentiment, first call resolution, average handle time, a digital resolution rate, digital containment rate. These are all different types of metrics or goals an organization could have.
But among the chief dos and don'ts is, make sure you're choosing AI that is specific to what your goals are. I would say very close second is making sure you're choosing AI that is purpose-built for customer experience. Or purpose-built for, if you're not in a contact center, whatever your specific type of organization does.
There are so many available artificial intelligence solutions right now, but it's really critical to choose AI that is designed and built on data that is specific to your organization. So in this instance, customer experience.
And that allows you to benefit from how those models and how that AI is built so that you can use something out of the box. You don't have to build everything on your own, because that could be very time-consuming. And also creates some ethical dilemmas if you don't have a large enough data set because your AI is only going to be as good as the data that it's trained upon. So you want to make sure it has as much data, and relevant data, for your use case as possible.
Laurel: So you did touch on this a little bit. Which is, how can AI and automation enhance the day-to-day work of contact center agents without creating additional challenges? How can it actually continuously improve both the employee and customer experience?
Michele: Yeah, of course. So I'll give a couple more examples. I think there is a few I gave earlier. So the first I think is just being objective about how a call has been handled, I think that's one of the most critical use cases.
And so at NICE, we have AI models that learn these different agent soft skills. So everything from how to ask good probing questions, to being empathetic, to taking ownership and resolving an issue efficiently. These models are looking at how to do that. And I think that's one of the pieces that helps in the day-to-day work for contact center agents. Because they are getting consistent feedback on how they're performing, but also the models continue to improve over time as well because you're giving the models new data to work from, new calls, new interactions. And then that is improving both the evaluations for the agents, but it's improving the customer experience as well.
Because if your baseline is that your sentiment level was at a five, and now you've expanded the baseline and you've increased your baseline and now you're at an eight, you're consistently improving in that way where you've now, one, measured what you want to do, which is improve customer experience. You've given your agents a measurement, a consistent measurement to deliver on your goal. And then three, you're continuing to measure over time as you have more different interactions.
So not only are your agents getting better, but your models become more finely-tuned for your organization as well.
Laurel: So as we're discussing this, in terms of coaching and training agents, how can AI-driven tools effectively provide that kind of real-time guidance without being intrusive? But then also, strike that balance between support and autonomy for the agents?
Michele: Yeah, and I think that's a great place to be thinking about. If you are a contact center agent, you are on the phone, and then you're also multitasking on your screen. You're looking for data, you're looking for information, you have the customer's card and hopefully information up from their previous interaction. You have maybe an IM message with your supervisor, you have a lot going on at one time.
So I think when you're thinking about things like real-time guidance, and coaching and training, this is where it becomes really crucial. I mentioned this being interaction-centric and having everything on one platform, but having the ability to use that sentiment data or customer satisfaction data in multiple places can be very powerful. Because then you're not introducing new information in real time.
I think that's the biggest piece to be aware of. Is that in real time, that is not the first time agents are seeing this information about how they could become more empathetic, or how they can deliver on their coaching that they had with their supervisor in a previous interaction.
So by putting everything, anchoring in on this interaction-centric piece and then converging everything on one type of a data platform. In the industry we call it CCaaS, contact center as a service. By delivering on one platform, you enable your organization to use the same data point in multiple places.
So the agent is using this data, they get a popup in real time. But they've also had conversations with their supervisor about these skill sets after their previous interactions. And it's that cycle, and it's that consistency, that makes agents better aware of and more adaptable for this environment. So that you're not going to them and giving them yet another thing that they need to resolve, but you're providing them with information that is relevant and real-time for that particular interaction that they're on.
Laurel: So you've touched on this here and there, but a key element of deploying any kind of new tool or technology is measuring its success. What metrics should organizations then prioritize to measure both customer satisfaction and operational efficiency?
Michele: Some of the key metrics that organizations most focus on in the contact center are net promoter score, so NPS score, or a customer satisfaction score. Those are key measurements of how a customer perceives the interaction. You'll also see things like customer sentiment, how a customer is feeling about the interaction, included in those measurements.
And then you get into some measurements that are more around the length of the call or efficiency-driven, like an average handle time or an average talk time. And I would say between the CSAT-type measurements and efficiency-type measurements, those make up the measurements for many of the voice types of interactions. So, how long a call is correlates directly to the cost of the call.
Then what's kind of exciting in this new space is, there's a lot more organizations that are moving into digital interactions as well. And organizations are looking at things like digital containment, or the number of digital resolutions. How many customer questions was my website or my chatbot able to resolve?
Those then translate into what could be cost savings for a voice interaction. Voice interactions are about a hundred times more expensive than a web or chatbot interaction. So by building effective chatbots, by building effective IVAs, they are also, in turn, improving their overall cost goals for their organization.
I'd say the other metric that everyone is focused on as well is agent retention. So are you giving your tools to your agents to support them in the coaching process and the quality process, in their interactions so that they have a better experience with your organization in answering questions, and that you're giving them tools to grow as well?
Being a contact center agent is probably one of the hardest and most difficult jobs in that business space. And they are on the phone, they're inundated with information. So any tools that you can provide them with to help them access information more quickly is hugely beneficial.
Laurel: So it's clear that there's lots of opportunities for greater efficiencies and optimizing customer experiences. But looking into the future, how do you see AI and customer experience evolving?
Michele: I think there's definitely going to be more use cases where we see ... And here at NICE, we're already integrating generative AI and conversational AI into our solutions. And as you adapt these new technologies, it's only going to build upon itself, where there's going to be more evolutions in this space.
I think one of the most exciting things that we've introduced recently is this idea of using generative AI. So we've put guardrails around it, and the guardrails are really crucial when you're working with artificial intelligence and the large language models, LLMs. We've all played with ChatGPT or Claude, and you can interact with those.
And what is really exciting that we've done is, we've used that type of technology to generate conversations and answers and information. But we've put guardrails on it so that organizations can better interact with just their customer experience specific data.
And what this means is, when you are in leadership in an organization, for example, if you were looking for a report, it may take you 12 emails multiple times back and forth saying what you'd like to see in that report. But if you have, again, all of these interactions on one platform, you've made it interaction-centric, you're using all these solutions that kind of compliment each other for every part of the interaction.
What you can do is, instead of emailing a data analyst back and forth for a report, you could interact with generative AI. You could type a question to say, "Hey, who are my top 10 performing agents by sentiment, and what are their key skills that they are using in those interactions?" Then you can generate a report based off of that.
What we're seeing is that all of these solutions are not necessarily replacing people, but we're seeing a lot of AI-adjacent or AI-augmented interactions in this contact center space that are coming into play.
And what this is doing is, it's allowing decision-makers to focus more on their overall strategy and the overall experience that they're delivering to customers. Rather than being very specific in emailing about a report, or even for agents to be able to type into a conversational AI interface that they can look for specific types of information, rather than searching everywhere for it.
So we are seeing a lot more AI augmented users. And so as everyone gets introduced to this technology, it's going to be those that are open to using new things and open to using AI, but also the ones that are selecting the right types of artificial intelligence to compliment their business that are going to be the most successful in using it, and gaining the efficiency and optimizing the customer experiences.
Laurel: That's great insight, Michele. Thank you so much for being on the Business Lab today.
Michele: Thanks so much, Laurel. It was great to be here.
Laurel: That was Michele Carlson, who is the senior product marketing manager at Nice, who I spoke with from Cambridge, Massachusetts, the home of MIT and MIT Technology Review.
That's it for this episode of Business Lab. I'm your host, Laurel Ruma. I'm the global director of Insights, the custom publishing division of MIT Technology Review. We were founded in 1899 at the Massachusetts Institute of Technology, and you can find us in print, on the web, and at events each year around the world.
For more information about us and the show, please check out our website at technologyreview.com. This show is available wherever you get your podcasts. If you enjoyed this episode, we hope you'll take a moment to rate and review us. Business Lab is a production of MIT Technology Review. This episode was produced by Giro Studio. Thanks for listening.
This content was produced by Insights, the custom content arm of MIT Technology Review. It was not written by MIT Technology Review’s editorial staff.
Keep Reading
Most Popular
Large language models can do jaw-dropping things. But nobody knows exactly why.
And that's a problem. Figuring it out is one of the biggest scientific puzzles of our time and a crucial step towards controlling more powerful future models.
How scientists traced a mysterious covid case back to six toilets
When wastewater surveillance turns into a hunt for a single infected individual, the ethics get tricky.
The problem with plug-in hybrids? Their drivers.
Plug-in hybrids are often sold as a transition to EVs, but new data from Europe shows we’re still underestimating the emissions they produce.
Stay connected
Get the latest updates from
MIT Technology Review
Discover special offers, top stories, upcoming events, and more.