The Ultimate Challenge For Recommendation Engines
The phrase “People who bought X, also bought Y” has become one of the celebrated monikers of the internet era. This particular form of words comes from recommendation engines that analyse the products you have bought in the past to suggest products you might like in future, usually based on the choices made by other people with similar tastes.
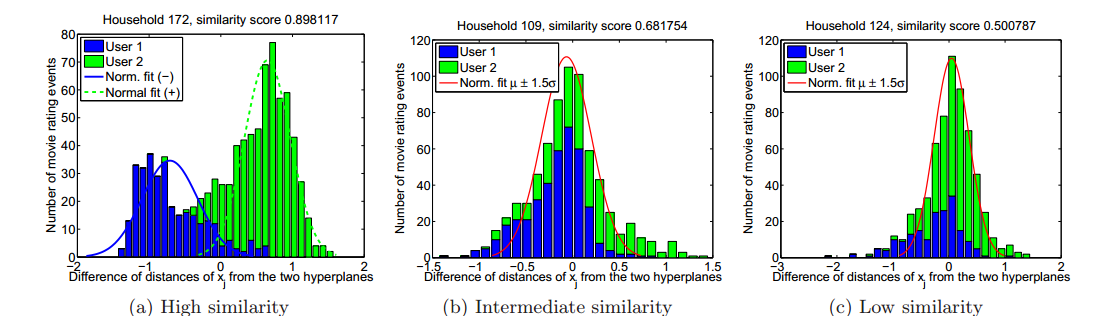
Good recommendation engines can increase sales by several percent. Which is why they have become one of the must-have features for online shops and services.
So it is not hard to understand why there is considerable interest in improving the performance of recommendation engines. Indeed, in 2006, the online movie provider, Netflix, offered a prize of $1 million to anybody who could improve their recommendation algorithm by more than 10 percent. The prize was duly snapped up a mere three years later.
So where might the next improvements come from?
Today, we get an answer of sorts thanks to the work of Amy Zhang at the Massachusetts Institute of Technology in Cambridge and a couple of pals. These guys point out that when it comes to online services such as movie providers, several individuals often share the same account. That means that the choice of movies and the ratings on this account are the combined choices of several different people.
The question they set out to answer is whether it is possible to identify shared accounts simply by studying the ratings associated with it. And if so, how should recommendations be modified in response?
They begin with two datasets of movie recommendations. The first consists of over 4 million recommendations from 171,000 users on over 20,000 movies. This dataset also has additional information about household arrangements for a subset of 600 users. Of these, 272 households have two users, 14 have three users and four have four users. So the recommendations from these households provide a ground truth of shared accounts.
Zhang and co also have the Netflix dataset of ratings by almost 500,000 users for over 17,000 movies.
They begin their analysis with a mathematical treatment of how to decompose a joint set of ratings into its component parts. The task is essentially one of finding a number of coherent clusters of recommendations that correspond to the number of people in the household.
In practice, this means finding clusters of similar movies with similar ratings. One technique that turns out to be important is that it is possible to allocate a few movies to different users with high confidence. For example, the movies Toy Story, Monsters Inc and Frozen may well have been watched and rated by a different individual than a cluster of movies including Texas Chainsaw Massacre, Alien and The Exorcist.
In mathematics, this is known as a subspace clustering problem and there are several standard approaches for solving it. Zhang and co first apply these methods to the datasets in which the households are known to find out which works best.
They then applied this method to around 55,000 users in the Netflix database who rated more than 500 movies. Their algorithm labelled 37,000 of these as single person accounts, 15,000 as 2-person accounts and 3000 as accounts used by 3 or more people.
There is no way of knowing whether this division is correct since the ground truth information is not available. However, it is possible to study these composite accounts to see whether they seem reasonable. “A visual inspection of the accounts that were labeled as composite yield some interesting observations,” say Zhang and co.
For example, they found in many accounts that sequels or seasons of the same TV show were grouped together. They also found that one user would prefer movies labelled as “Science Fiction and Fantasy” while another might prefer movies labelled as “Romantic”. That seems to give Zhang and co confidence that their algorithm is on the right track.
The final question address is how to change recommendations once the algorithm has established that more than one user shares the same account. The answer is straightforward. Simply display the top recommendations for each user.
That’s an interesting approach although it is not clear just how much better these recommendations perform over conventional engines in terms of whether they increase sales or not. That is an obvious goal for future research.
Interestingly, Zhang and co point out that this approach may allow a single person to appear as a composite by deliberately including ratings on films that they would not normally like. “Altering or augmenting one’s rating profile to appear as a composite user, with the purpose of obscuring, for example one’s gender, is an interesting research topic,” they say.
Perhaps we will see the results of this research at some point in the future.
Ref: arxiv.org/abs/1408.2055 : Guess Who Rated This Movie: Identifying Users Through Subspace Clustering
Keep Reading
Most Popular
Large language models can do jaw-dropping things. But nobody knows exactly why.
And that's a problem. Figuring it out is one of the biggest scientific puzzles of our time and a crucial step towards controlling more powerful future models.
How scientists traced a mysterious covid case back to six toilets
When wastewater surveillance turns into a hunt for a single infected individual, the ethics get tricky.
The problem with plug-in hybrids? Their drivers.
Plug-in hybrids are often sold as a transition to EVs, but new data from Europe shows we’re still underestimating the emissions they produce.
Stay connected
Get the latest updates from
MIT Technology Review
Discover special offers, top stories, upcoming events, and more.