Extracting Meaning from Millions of Pages
A software engine that pulls together facts by combing through more than 500 million Web pages has been developed by researchers at the University of Washington. The tool extracts information from billions of lines of text by analyzing basic relationships between words.
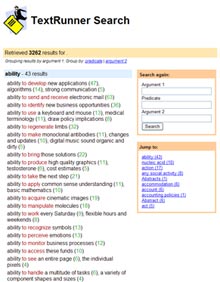
Some experts say that this kind of “automated information extraction” will likely form the basis for far more intelligent next-generation Web search, in which nuggets of information are first gleaned and then combined intelligently.
The University of Washington project represents a scaling up of an existing technology developed there called TextRunner in terms of both the number of pages and the scope of topics that it can analyze.
“The significance of TextRunner is that it is scalable because it is unsupervised,” says Peter Norvig, director of research at Google, which donated the database of Web pages that TextRunner analyzes. “It can discover and learn millions of relations, not just one at a time. With TextRunner, there is no human in the loop: it just finds relations on its own.”
Norvig explains that previous technologies have required more guidance from the programmer. For example, to find the names of people who are CEOs within millions of documents, you’d first need to train the software with other examples, such as “Steve Jobs is CEO of Apple, Sheryl Sandberg is CEO of Facebook.” Norvig adds that Google is doing similar work and is already using such technology in limited contexts.
TextRunner gets rid of that manual labor. A user can enter, for example, “kills bacteria,” and the engine will come up with of pages that offer the insights that “chlorine kills bacteria” or “ultraviolet light kills bacteria” or “heat kills bacteria”–results called “triples”–and provide ways to preview the text and then visit the Web page that it comes from.
The prototype still has a fairly simple interface and is not meant for public search so much as to demonstrate the automated extraction of information from 500 million Web pages, says Oren Etzioni, a University of Washington computer scientist leading the project. “What we are showing is the ability of software to achieve rudimentary understanding of text at an unprecedented scale and scope,” he says.
Etizioni says TextRunner’s ability to extract meaning quickly and at huge scale flowed from his group’s discovery of a general model for how relationships are expressed in English that holds true no matter the topic. “For example, the simple pattern ‘entity1, verb, entity2’ covers the relationship ‘Edison invented the light bulb’ as well as ‘Microsoft acquired Farecast’ and many more,” he says. “TextRunner relies on this model, which is automatically learned from text, to analyze sentences and extract triples with high accuracy.”
TextRunner also serves as a starting point for building inferences from natural-language queries, which is what the group is now working on. To give a simple example: if TextRunner finds a Web page that says “mammals are warm blooded” and another Web page that says “dogs are mammals,” an inference engine will produce the information that dogs are probably warm blooded.
This is analogous to technology developed by Powerset, which was acquired by Microsoft last year. Shortly before this acquisition, Powerset unveiled a tool that was limited to extracting facts from only about two million Wikipedia pages. The TextRunner technology handles Wikipedia pages plus arbitrary text on any page, including blog posts, product catalogues, newspaper articles, and more.
“This line of work has been making important advances in the scale at which these tasks can be approached,” says Jon Kleinberg, a computer scientist at Cornell University who has been following the University of Washington search research. He added that “this work reflects a growing trend toward the design of search tools that actively combine the pieces of information they find on the Web into a larger synthesis.”
Keep Reading
Most Popular
Large language models can do jaw-dropping things. But nobody knows exactly why.
And that's a problem. Figuring it out is one of the biggest scientific puzzles of our time and a crucial step towards controlling more powerful future models.
How scientists traced a mysterious covid case back to six toilets
When wastewater surveillance turns into a hunt for a single infected individual, the ethics get tricky.
The problem with plug-in hybrids? Their drivers.
Plug-in hybrids are often sold as a transition to EVs, but new data from Europe shows we’re still underestimating the emissions they produce.
Stay connected
Get the latest updates from
MIT Technology Review
Discover special offers, top stories, upcoming events, and more.