Human Model Completed
Researchers at the University of California, San Diego, have constructed the first complete computer model of human metabolism. Available free on the Web, the model is a major step forward in the fledging field of systems biology, and it will help researchers uncover new drug pathways and understand the molecular basis of cancer and other diseases.
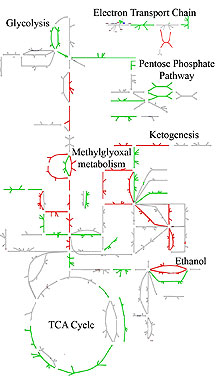
Metabolism is the sum of all chemical reactions involved in breaking down the nutrients in food into energy and using them as the raw materials for making everything the body needs, from hormones like insulin to the lipids that make up cell membranes. The computer model of metabolism, constructed by researchers in the lab of Bernhard Palsson, professor of bioengineering, connects all such known chemical reactions in the body to every human gene.
Similar models of metabolism in microbes such as yeast and E. coli–including several generated by Palsson’s lab–are enabling researchers to engineer organisms that more efficiently produce products like ethanol and antimalarial drugs. (See “Watching Bacteria Evolve in the Lab” and “Engineered Microbes Boost Ethanol.”) But, says Aviv Regev, a computational biologist at the Broad Institute, in Cambridge, MA, “a high-quality model of metabolism in humans has been elusive.”
The new model includes every known gene and every metabolic reaction Palsson’s group uncovered in an extensive search of the scientific literature. A given gene in the database is associated with its protein product, which might be associated with a number of metabolic reactions, which are associated with other reactions, which are associated with nutritional inputs like glucose and the output of energy or a product like melatonin. Palsson calls the model “a mathematical representation of all this data.” The online database will be updated continuously.
Regev says that one of the model’s most promising applications is as a “scaffold on which to project data” from gene-expression profiling studies. Using microarrays, it is now possible to find differences in gene expression between, for example, normal liver tissue, cancerous liver tissue, and diabetic liver tissue. Such data has the potential to reveal both the molecular causes of disease and potential drug targets. But in these studies, says Regev, “changes in gene expression are spread all over [the genome].”
Using the model, researchers can input which genes are expressed in a diseased tissue and get as an output the metabolic pathways in which these genes are involved–as opposed to painstakingly searching the scientific literature for information one gene at a time. For example, in the case of the liver, the model might tell researchers that a gene overexpressed in cancerous liver tissue is involved in specific metabolic reactions, creating particular products. Researchers might then look for a drug that targets these pathways or products. “There are very large-scale metabolic shifts in cancer tissue,” Regev says.
James Collins, professor of biomedical engineering at Boston University, has already begun using network-level approaches to understanding cancer, and he says he will use Palsson’s model in his research. “You can look at differentially expressed genes in a patient with prostate cancer,” says Collins. “Among those, are there pathways that indicate the underlying processes of the disease?” It will “enable us to filter and condense complex data and identify drug targets.”
What’s more, the model could help researchers better understand and optimize existing drugs. “It’s difficult to figure out which genes are affected indirectly by a drug,” says Collins. “You want to know what you’re hitting to get better chemistry, stronger intellectual property, and understand side effects.” Palsson points out that because the network can identify multiple ways to generate the same outcome, it may help drug companies come up with compounds that have the same effects–alternatives to statin drugs like Lipitor, for example–without violating their competitors’ patents.
Keep Reading
Most Popular
Large language models can do jaw-dropping things. But nobody knows exactly why.
And that's a problem. Figuring it out is one of the biggest scientific puzzles of our time and a crucial step towards controlling more powerful future models.
How scientists traced a mysterious covid case back to six toilets
When wastewater surveillance turns into a hunt for a single infected individual, the ethics get tricky.
The problem with plug-in hybrids? Their drivers.
Plug-in hybrids are often sold as a transition to EVs, but new data from Europe shows we’re still underestimating the emissions they produce.
Stay connected
Get the latest updates from
MIT Technology Review
Discover special offers, top stories, upcoming events, and more.